Veith Weilnhammer
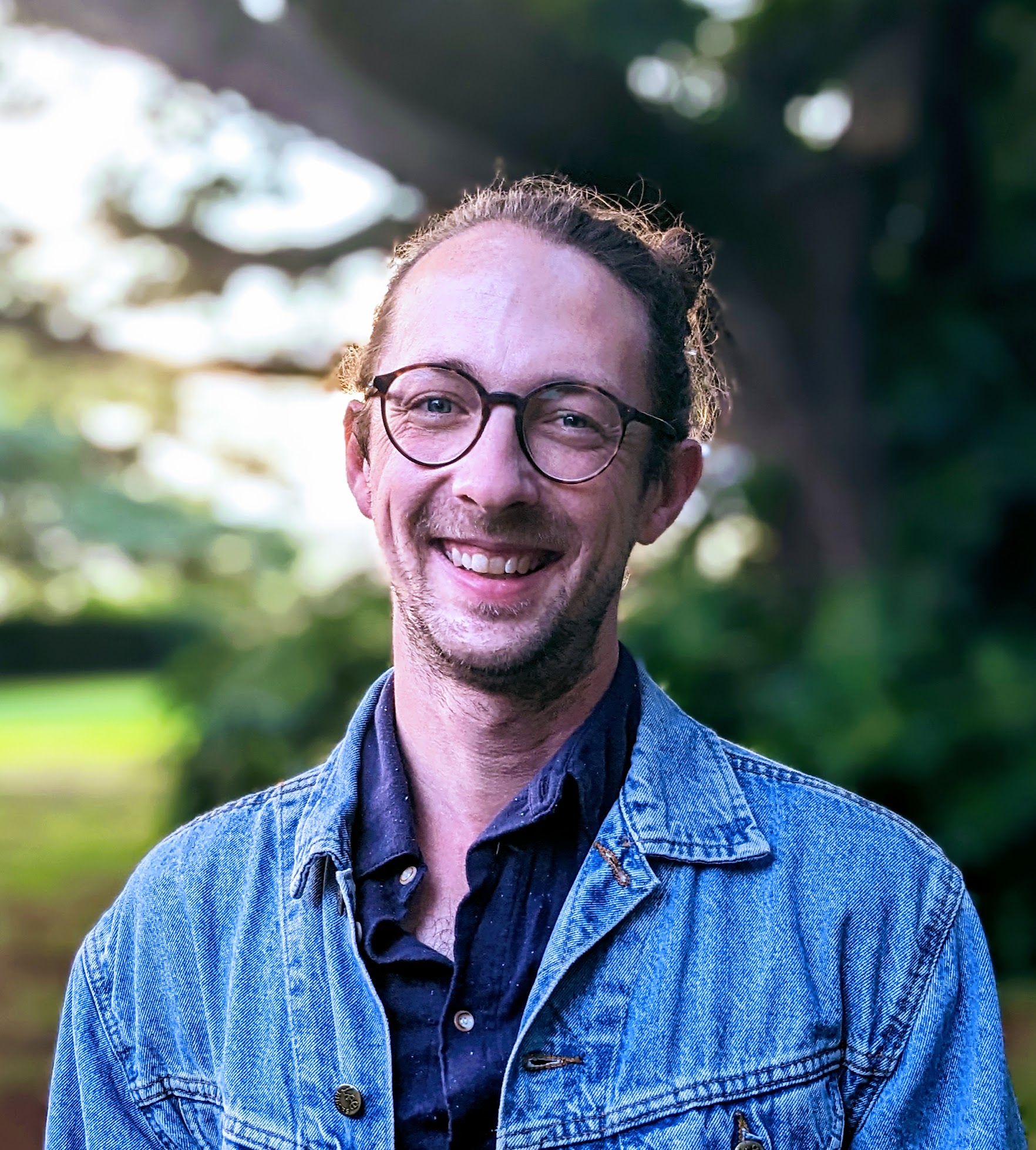
I am a neuroscientist, psychiatrist, and psychotherapist. I work on digital technologies to better understand, predict, and improve mental health.
selected publications
- N-methyl-d-aspartate receptor hypofunction causes recurrent and transient failures of perceptual inferenceVeith Weilnhammer, Marcus Rothkirch, Deniz Yilmaz, and 6 more authorsBrain, Jan 2025
Perception integrates external sensory signals with internal predictions that reflect prior knowledge about the world. Previous research suggests that this integration is governed by slow alternations between an external mode, driven by sensory signals, and an internal mode, shaped by prior knowledge. Using a double-blind, placebo-controlled, cross-over experiment in healthy human participants, we investigated the effects of the N-Methyl-D-aspartate receptor (NMDAR) antagonist S-ketamine on the balance between external and internal modes. We found that S-ketamine causes a shift of perception toward the external mode. A case-control study revealed that individuals with paranoid Scz, a disorder repeatedly associated with NMDAR hypofunction, spend more time in the external mode. This NMDAR-dependent increase in the external mode suggests that the symptoms of schizophrenia are caused by recurring dissociations of perception from prior knowledge about the world.
- Dynamic predictive templates in perceptionVeith Weilnhammer, Yuki Murai, and David WhitneyCurrent Biology, Aug 2024
Hallucinations are vivid and transient experiences of objects, such as images or sounds, that occur in the absence of a corresponding stimulus. To understand the neurocomputational mechanisms of hallucinations, cognitive neuroscience has focused on experiments that induce false alarms (FAs) in healthy participants, psychosis-prone individuals, and patients diagnosed with schizophrenia. FAs occur when participants make decisions about difficult-to-detect stimuli and indicate the presence of a signal that was, in fact, not presented. Since FAs are, at heart, reports, they must meet two criteria to serve as an experimental proxy for hallucinations: first, FAs should reflect perceptual states that are characterized by specific contents (criterion 1). Second, FAs should occur on a timescale compatible with the temporal dynamics of hallucinations (criterion 2). In this work, we combined a classification image approach with hidden Markov models to show that FAs can match the perceptual and temporal characteristics of hallucinations. We asked healthy human participants to discriminate visual stimuli from noise and found that FAs were more likely to occur during an internal mode of sensory processing, a minute-long state of the brain during which perception is strongly biased toward previous experiences (serial dependency). Our results suggest that hallucinations are driven by dynamic predictive templates that transform noise into transient, coherent, and meaningful perceptual experiences.
- Sensory processing in humans and mice fluctuates between external and internal modesVeith Weilnhammer, Heiner Stuke, Kai Standvoss, and 1 more authorPLOS Biology, Aug 2023
Perception is known to cycle through periods of enhanced and reduced sensitivity to external information. Here, we asked whether such slow fluctuations arise as a noise-related epiphenomenon of limited processing capacity or, alternatively, represent a structured mechanism of perceptual inference. Using 2 large-scale datasets, we found that humans and mice alternate between externally and internally oriented modes of sensory analysis. During external mode, perception aligns more closely with the external sensory information, whereas internal mode is characterized by enhanced biases toward perceptual history. Computational modeling indicated that dynamic changes in mode are enabled by 2 interlinked factors: (i) the integration of subsequent inputs over time and (ii) slow antiphase oscillations in the impact of external sensory information versus internal predictions that are provided by perceptual history. We propose that between-mode fluctuations generate unambiguous error signals that enable optimal inference in volatile environments.
- An active role of inferior frontal cortex in conscious experienceVeith Weilnhammer, Merve Fritsch, Meera Chikermane, and 5 more authorsCurrent Biology, Jul 2021
In the search for the neural correlates of consciousness, it has remained controversial whether prefrontal cortex determines what is consciously experienced or, alternatively, serves only complementary functions, such as introspection or action. Here, we provide converging evidence from computational modeling and two functional magnetic resonance imaging experiments that indicated a key role of inferior frontal cortex in detecting perceptual conflicts caused by ambiguous sensory information. Crucially, the detection of perceptual conflicts by prefrontal cortex turned out to be critical in the process of transforming ambiguous sensory information into unambiguous conscious experiences: in a third experiment, disruption of neural activity in inferior frontal cortex through transcranial magnetic stimulation slowed down the updating of conscious experience that occurs in response to perceptual conflicts. These findings show that inferior frontal cortex actively contributes to the resolution of perceptual ambiguities. Prefrontal cortex is thus causally involved in determining the contents of conscious experience.